An Introduction to Data Equity
What is Data Equity?
Data equity is a term we use to describe an ethos toward using data for health, well-being, and equity. Data equity recognizes the importance of considering issues of power, bias, and discrimination in data collection, analysis, and interpretation. This includes seeking community ownership of data, applying practices that ensure protection and power-sharing for people and communities, recognizing and seeking to correct legacies of discrimination through data, recognizing the dangers of a single story, and weaving data and storytelling to tell a more complete picture. Ultimately, advancing data equity helps build community power and emphasizes the need to address systemic inequities and power imbalances.
Data equity requires balancing the potential benefits and risks of different data practices in specific contexts. It involves ensuring the benefits and risks associated with data use are distributed justly, which can help prevent biases and inequalities, and lead to better health outcomes for all. Data equity is important for identifying and addressing health disparities and social determinants of health in a fair and just manner.
Data equity is not just an ethical imperative, it leads to better data and better well-being outcomes. Data equity:
Results in better quality data;
Builds belonging and civic muscle;
Improves health, well-being, and equity; and
Adds sustainability and long-term community partnership.
In this post, we outline several key dimensions of data equity:
Community data for community power
Data about people and communities
Community Data for Community Power
Even centuries later, the saying “Knowledge is power” (based on the writing of Sir Francis Bacon, 1597) perfectly captures the timeless truth that shared knowledge is a cornerstone of reputation and influence—and thus power. Today, we look to the data for that knowledge. The data help us describe—and quantify—the world around us. The data give us the information we need for public decision-making, policy development, resource allocation, and advocacy. Data are power.
Community data help build community power by fueling advocacy. Advocates often rely on data about community strengths, assets, opportunities, and needs to inform their position and make their case. Data can lend authority and legitimacy to community-based and grassroots efforts and may spark or give shape to social movements.
Community ownership of data builds civic capacity, belonging, and civic muscle, and shifts power toward communities--importantly marginalized communities and communities of color. Community ownership refers to data that are owned and controlled by the community from which they were collected. When communities own their data, they gain control over their information and how the data are collected, used, and shared. Community ownership of data:
Promotes community self-determination;
Builds community trust and fosters stewardship and collaboration;
Addresses information asymmetry;
Ensures data collection and analysis has cultural relevance and context; and
Sustainably builds local capacity and fosters long-term engagement.
How Community-Based Organizations Use Data to Advance Racial Equity
Resource - Blog
Brought to you by Urban Institute
Native Data Sovereignty Can Address Data Gaps and Improve Equity
Resource - Blog
Brought to you by Urban Institute
Published on 06/13/2022
Inclusive Data Systems
Establishing inclusive data systems is important to build community power, and prevent discriminatory use of data and other data misuse. Data have been used to perpetuate inequality and discrimination throughout history. For example, data were used to drive discriminatory policies like redlining in the 1930s, when the Federal Housing Administration declared that no loan could be “economically sound” if the property was located in a primarily-Black neighborhood. With recent technological advances in machine learning, artificial intelligence, and other fields, new opportunities for data analysis have emerged. However, there are concerns that these advancements may lead to biased and discriminatory use of data. Inequitable use of data can lead to inaccurate reporting and poor decision-making, unintended consequences, and loss of trust. To promote equity and justice, it is crucial that we recognize issues of data privacy and prevent data from being weaponized against communities.
Shifting a community's orientation toward knowledge production is an inclusive way to shift community power. “Nothing about us without us” is a powerful mantra that refers to the need to partner with the community on research and data collection activities occurring within the community. Community-based participatory research (CBPR) is one approach, in which researchers, organizations, and community members collaborate on all aspects of a research project. Key principles of CBPR include: (1) recognizing the community as a unit of identity, (2) building on community strengths and resources, (3) promoting co-learning, and (4) balancing research and action in ways that mutually benefit science and the community. Community ownership of data, CBPR, engaging community members in data collection, sharing, and analyses, and other leading practices are helping to correct for legacies that have oppressed and exploited communities in the name of data and research.
Do No Harm Guide: Additional Perspectives on Data Equity
Resource - Report
Brought to you by Urban Institute
A Toolkit for Centering Racial Equity Throughout Data Integration
Tool - Toolkit/toolbox
Brought to you by University of Pennsylvania
Data About People and Communities
Data help describe our world—data tell us about people and communities and shed light on the vital community conditions that give rise to health and well-being. We often use demographic data (e.g., age, gender, race, ethnicity) to measure health and well-being conditions and outcomes. Using multiple kinds of data—qualitative and quantitative, and story—balances narratives and gives nuance to our understanding of community conditions.
Access to the vital community conditions and health and well-being outcomes differ across place and population. Disaggregating data by geography (e.g., county, neighborhood) and demographic group (e.g., age, gender, race, and ethnicity) helps identify underlying patterns of variation by place and population. Disaggregating data refers to the process of breaking down aggregated data into smaller informational units in order to examine a characteristic or dimension. For example, we can break down or disaggregate health behavior or outcome data, such as cancer incidence or receiving a flu shot, by race and ethnicity to examine issues of health access and opportunity in communities of color. Disaggregated data help uncover disparities, identify trends, inform policies and programs, and expose systems of privilege and oppression.
Despite its benefits, disaggregating data also has some risks. Disaggregated data can reinforce stereotypes and perpetuate harmful narratives about specific groups and communities. There are real people behind the data, and it’s important to use humanizing language when discussing people and communities. Disaggregated data can also create privacy concerns when populations are small in size and obscure issues of intersectionality--the acknowledgment that everyone has their own unique experiences of discrimination and oppression, and we must consider everything and anything that can marginalize people, such as gender, race, class, sexual orientation, and physical ability.
Counting a Diverse Nation: Disaggregating Data on Race and Ethnicity to Advance a Culture of Health
Resource - Report
Brought to you by PolicyLink
Five Ethical Risks to Consider before Filling Missing Race and Ethnicity Data
Resource - Report
Brought to you by Urban Institute
Privacy and Confidentiality
Data about people and communities introduce concerns about privacy and confidentiality and require implementing practices that protect the people behind the data.
Data privacy refers to protection and control of an individual's personal information or data. Individuals have rights with regard to how personal data are collected, used, stored, and shared by organizations or entities. The HIPAA Privacy Rule gives patients an array of rights to their protected health information. HIPAA and other standards for data privacy seek to ensure that personal information is protected while allowing for the flow of that information for legitimate purposes. The following practices are used to protect data privacy:
Informed consent ensures research participants and patients have full information upon which to make autonomous decisions relating to their study participation or treatment
De-identifying health information decouples health and identifying information
Data suppression suppresses data for small populations
Data confidentiality deals with issues of access to data. Confidentiality refers to protecting sensitive or confidential information from unauthorized access, disclosure, or use. Confidentiality seeks to ensure that only authorized individuals or entities can access and handle the data in question. This helps to protect people and communities by preventing their data from falling into the wrong hands. The following are practices used to protect data confidentiality:
Restricted access limits access to sensitive data to only those who need them
Data encryption encrypts sensitive data to protect them from unauthorized use or interception
Confidentiality agreements define the responsibilities and obligations of those handling sensitive data, and the consequences of unauthorized access, disclosure or misuse
Data masking and anonymization involves replacing, removing, or altering sensitive data to ensure individuals cannot be identified
Current standards for data privacy and confidentiality were born out of a legacy of harms. The United States Public Health Service Syphilis Study in Tuskegee/Macon County, Alabama, 1932-1972 is a potent example of unethical research practices. The study observed the natural progression of syphilis in black men by withholding treatment, even after an effective cure became available. The study participants were deceived and denied informed consent, resulting in severe health consequences. This atrocity and others committed in the name of research contribute to mistrust of medical and research institutions. While advances in data privacy and confidentiality serve to protect against future harms, people and communities have good reason to distrust the data, and the people collecting and disseminating it.
Confidentiality Toolkit
Resource - Guide/handbook
Brought to you by Administration for Children and Families
Data Access and Sharing
High standards for data privacy and confidentiality set the stage for making data accessible more broadly. Data sharing is the act of making data available to people, organizations, stakeholders, or other partners. Sharing data with the community and stakeholders enables better understanding of complex issues and can inform collaborative decision-making. It promotes collaboration, transparency, and accountability that help us work better together and build trust needed for long-term, sustainable change. Through data sharing, we can cultivate a culture of shared stewardship and community ownership of data, and power movements that transform systems for health, well-being, and equity.
Data democratization refers to making data accessible to people and assuring they have what they need to understand the data. Data literacy is a related concept that deals with the knowledge and skills to understand and use data. Through data literacy and sharing, we can promote more equitable community data systems and a vibrant data democracy.
Data Democratization: The Unsung Hero of Health Equity
Resource - Blog
Brought to you by Health Leads
Connecting the Dots: A Data Sharing Framework for the Local Public Health System
Resource - Policy Brief
Brought to you by NACCHO
Practicing Data Equity
Those working to advance health equity have a duty to also promote and practice data equity. Principles for Using Public Health Data To Drive Equity outlines principles to guide data practice:
Recognize and define systemic factors
Allow for cultural modification
Create shared data agreement
Use equity-mindedness for language and action
Facilitate data sovereignty
Stewards and changemakers can advance data equity in a number of ways:
Shift to practices where communities participate in the research process as much as possible and own their own data. Importantly, community members ought to be compensated for their time, expertise, and resources for all research and data collection work.
Ensure that data are easily available and accessible to stakeholders and community members is important. Using user-friendly platforms for data exploration can aid in this, as can providing clear documentation around data sources, collection methods, and methodologies.
Invest in data infrastructure and capacity building, including allocating resources to build robust data infrastructure, including data collection, storage, and analysis systems
Use Data visualization to make data accessible to a wide range of audiences. Visual representations of data can be better understood by people of different ages, cultures, and educational backgrounds, aiding in data sharing and stakeholder engagement because it enables people to quickly grasp complex data, understand meaning and implications, make informed decisions, and communicate findings to others.
Engage communities and stakeholders: Regardless of whether you’re examining quantitative or qualitative data, data equity can be built by simply “checking in” with people with lived experience to ensure key takeaways are consistent with real-life experience and to discover implications that may not be apparent in data results. Leaders should actively engage communities, seek input, and incorporate diverse perspectives in data-driven initiatives. Engaging communities ensures that data initiatives are responsive to local needs, values, and priorities, fostering a sense of ownership and trust.
Address potential data biases and disparities: Ensure data collection processes are inclusive and representative; invest in mitigating biases in algorithms and machine learning systems that can perpetuate existing inequalities.
Ensure data privacy and security: prioritize protecting individual privacy and maintaining data security while advancing data equity.
Advocate for equitable policies and regulations that promote data equity. This includes advocating for equal access to data, against discriminatory practices, and pushing for regulations that protect individual rights and promote fair and responsible data use.
Principles for Using Public Health Data To Drive Equity
Resource - Report
Brought to you by CDC Foundation
Related Topics
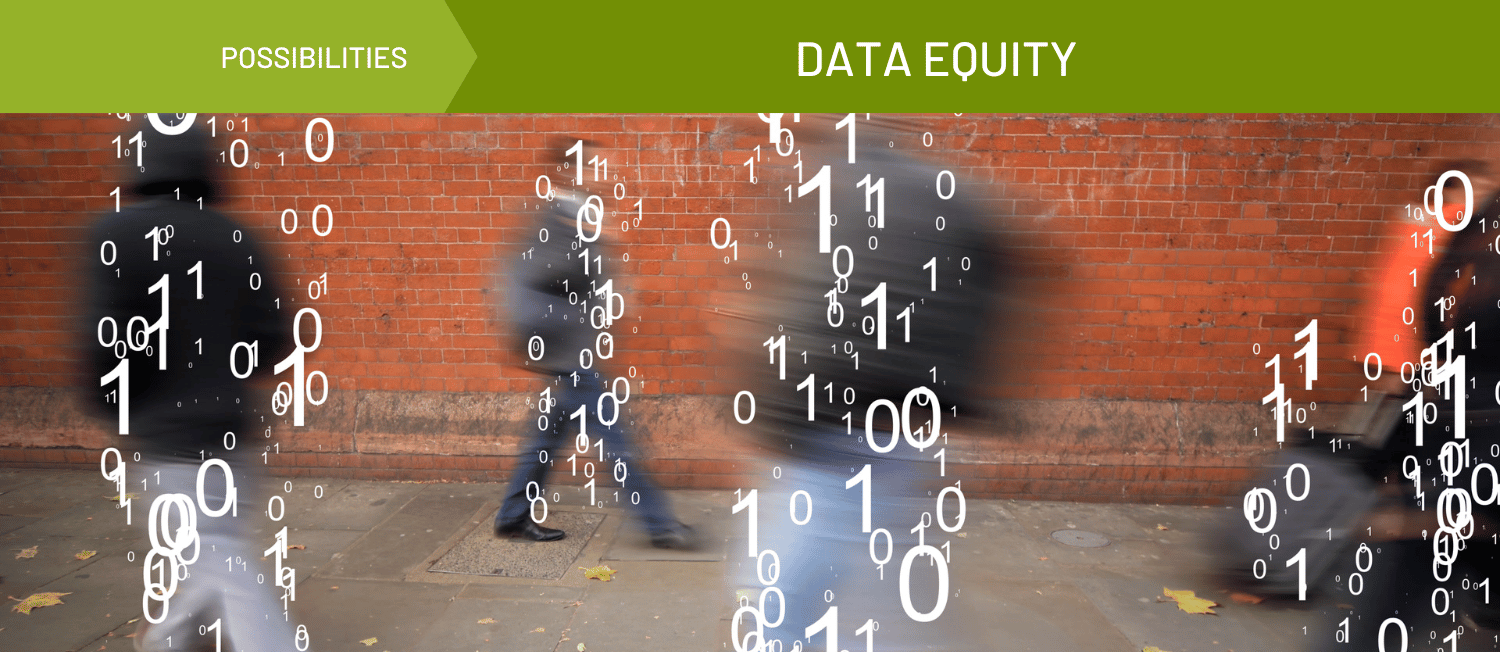